|
DOQS SIX SIGMA SERIES
Design for Six Sigma II
The rigor established in DFSS allows the design process to look at details
and opportunities otherwise unavailable to solution design teams. This
course covers the creative concept generation techniques of Six Sigma,
and the detailed decomposition and experimental techniques needed to define
optimal solutions across a variety of design dimensions and concerns that
have been mapped to a complete and prioritized voice-of-the-customer.
This course results in learners reaching the level required of DFSS Green
Belt certification in many organizations. (4 days, $20,000,
Prerequisite: Design for Six Sigma I)
Process Coverage
Design for Six Sigma is not independent of the Six Sigma Improvement
model. Opportunities for new systems, or components of systems, arise
out of the DMAIC analysis process. Implementing a system using DFSS always
takes place in the context of the DMAIC-driven improvement and always
results in an implementation that is sustained by DMAIC-derived controls,
even if the DMAIC efforts are not explicitly associated with the DFSS
effort.
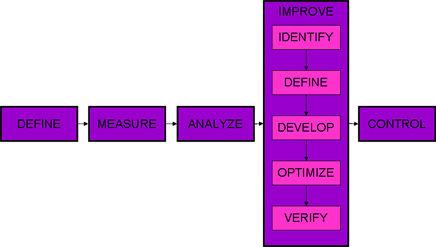
Lifecycle Coverage (DFSS)
Design for Six Sigma is based on an Identify-Define-Develop-Optimize-Verify
(IDDOV) lifecycle that emphasizes identifying and designing innovative
solutions against opportunities defined during traditional DMAIC initiatives.
The DMAIC lifecycle is viewed as the driver of scope and direction.
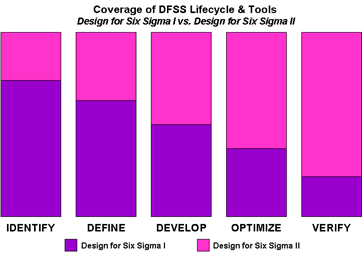
This course covers the entire IDDOV lifecycle, emphasizing
detailed activities that were omitted from the Design for Six Sigma I
course.
-
Identify the Opportunity - Driven by the measurement
and analysis phases of DMAIC, identification activities clarify particular
product or service opportunities that can be implemented to help solve
the problems being addressed by the DMAIC activity. Whether the need
is for a comprehensive systems solution, or a collection of independent
components that collectively will solve the problem, their identification
initiates the DFSS process.
-
Define the Requirements - Comprehensive requirements
engineering is a central theme of DFSS, with integrated models developed
for Customer Needs, Business Processes, Functional Systems, Systems
Designs, and Operational Support. Emphasis is placed on defining exhaustive
requirements with a high level of control and quantification. Customer
needs define requirements priorities, leading to a requirements management
model that might implement as a single solution, or a series of releases
planned over time.
-
Develop the Concept - As requirements progress toward
implementation details, various conceptual solutions are continuously
being identified and evaluated as stronger and stronger alternatives
emerge and drive subsequent engineering activities. Evaluation might
include various forms of experimentation, simulation, or prototyping
to better understand design parameters and performance relationships.
-
Optimize the Design - Once a best conceptual solution
has solidified in response to the prioritized and allocated requirements,
the design parameters and performance relationships are driven toward
optimal values using further experimentation and analysis. Critical
design variables and functional dimensions are designed toward maximizing
satisfaction and performance.
-
Verify Conformance - Once a systematic and rigorous
design has been optimized, it must be verified and validated against
the original requirements, as well as the critical-to-quality control
values that have been established at each level of the overall design.
The quantification of requirements must be assured, followed by the
review and approval of stakeholders to be impacted by the design.
Other common DFSS lifecycle models are also discussed, including Define-Measure-Analyze-Design-Verify
(DMADV) and Define-Model-Optimize-Verify-Control (DMOVC). These lifecycles
aim to accomplish goals similar to those of the IDDOV lifecycle. They
are typically seen in environments trying to implement DFSS on a standalone
basis, independent of DMAIC. We believe that DFSS works best if it attempts
to integrate the findings and tools of DMAIC into a comprehensive design.
DMAIC measurement and analysis activities always lay the groundwork for
effective design, and so we integrate them to illustrate and maximize
that effect.
Tool Coverage
The tools in this course are all discussed in the broader context of
the 4-tier Quality Function Deployment (QFD) model covered in Design for
Six Sigma I course:
-
KANO Analysis - Collecting requirements using the
voice of the customer often leaves two extreme categories of requirements
missing or under represented. KANO Analysis characterizes additional
requirements as delighters or dissatisfiers; the former representing
opportunities to delight customers with unexpected functions and features,
and the latter opportunities to recognize that many customer expectations
go beyond the requirements that have been made explicit.
-
Triz Concept Selection - The Theory of Inventive
Problem Solving (TRIZ) provides a creative approach for identifying
creative and unexpected solutions to problems and design challenges
that otherwise appear insurmountable. The 40 principles in TRIZ provide
a shopping list of heuristics, one of which will illuminate a creative
and relevant solution to virtually any design challenge.
-
Design for "X" - Any number of factors
might be relevant to the definition of quality during a systems initiative.
DFSS involves being able to incorporate a variety of factors "X"
into a design, working toward a solution set that optimizes their
interaction against customer needs and requirements. The "X"
factors covered in this course include Flexibility, Efficiency, Useability,
Portability, Reusability, and Scaleability. (Note: Testability, Reliability,
and Maintainability were covered in Design for Six Sigma I.)
-
Design of Experiments (DOE) - Effectively designed
experiments to define the relationships among key design parameters
is essential to identifying optimal design solutions on multiple levels
and scale of design.
-
Conjoint Analysis - When a range of possible design
options is evaluated through a weighted analysis customer opinions
and reactions to those design options, the relative worth of each
individual design option can be calculated. Known as Conjoint Analysis
- a specialized form of Design of Experiments - such analysis offers
a cost-effective way to quickly prioritize a collection of non-exclusive
design parameter settings.
-
Monte Carlo Analysis - Complex systems exhibit nonlinear
behaviors because of the interaction of many variables, each of which
varies within statistically predictable boundaries. Monte Carlo Analysis
provides a simulation of key interactions based on the inherent probabilities
associated with each variable in order to predict the frequency and
variability of extreme or peak interactions. These simulations are
used to ensure that worst case design conditions are accounted for
in system designs so that rare interactions do not result in system
failures.
-
Dashboards - In order to design systems that are
self-aware and capable of self-correction, internal metrics must be
made visible to system processes and controls. Collectively, such
data defines a dashboard. Designing dashboards requires an analysis
of critical metrics and the transfer functions that relate them.
-
Scorecards - To integrate the designed system into
the broader Control Plan associated with the initiating DMAIC effort,
critical metrics associated with critical to quality customer requirements
must be made available for monitoring and control. Collectively, such
metrics constitute a scorecard. Effective scorecard design builds
upon the included dashboards and transfer functions while being guided
by the pattern of design variables that tie back more directly to
original customer requirements.
|